The State of Speech-to-Text Applications Today
Modev News VOICE Summit 22-08-1 Modev Staff Writers 5 min read
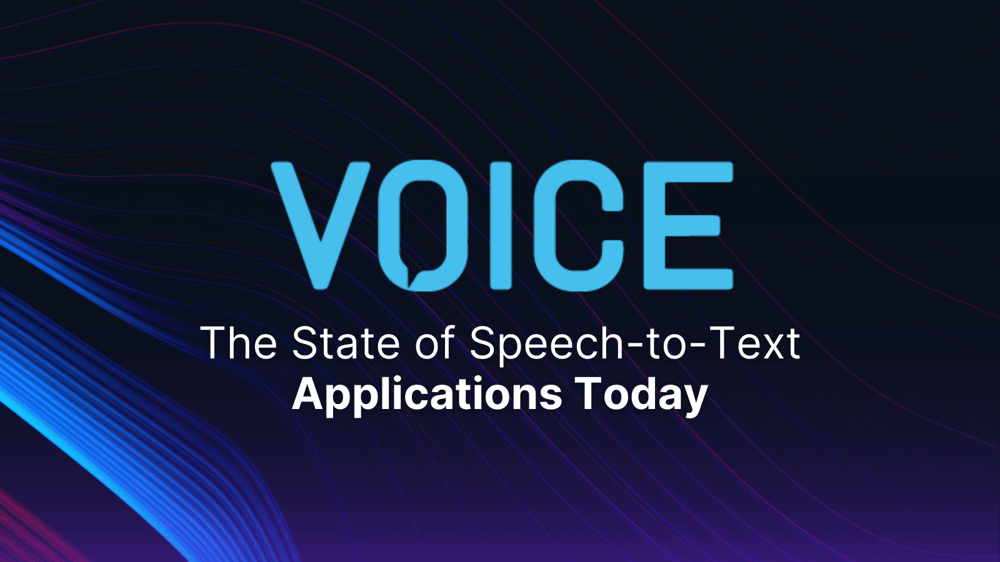
Speech-to-transcription applications have been with us for quite a while. The first speech recognition system was actually built in 1952 by Bell Laboratories. The system was called "Audrey" and could recognize the utterances of numbers zero to nine with over 90% accuracy. That may not sound all that impressive to us today, but in 1952, it was pretty amazing.
But that also highlights my point, we know that speech recognition - and by extension, speech-to-text - has come a long way and can interpret much more than numbers. But what exactly is the state of speech-to-text today? With all the recent advancements in AI-driven tech in general, and voice tech specifically, surely some of that has spilled over to and enhanced speech-to-text applications.
Indeed it has. This post looks at the state of speech-to-text applications today, the role AI has played in its development, and some of the challenges speech-to-text applications face today and will hopefully overcome in the near term.
AI, ML, and NLP
By now, most of us have heard these "buzzwords," - and they're all associated with speech-to-text applications. Artificial intelligence (AI), machine learning (ML), and natural language processing (NLP) are what opened the door to speech-to-text applications’ expanded use today. But while these terms are sometimes used interchangeably, they are three different things.
- Artificial intelligence (AI) is more of an umbrella term designating the field in computer science that aims to develop "intelligent" software with similar problem-solving skills to a human being. AI uses ML and NLP in its endeavor to achieve that goal.
- Machine learning (ML) is a component of AI research that uses statistical models and massive troves of data to "teach" computers to perform complex, judgment-based tasks like speech-to-text.
- Finally, natural language processing (NLP) is another component of AI research directly related to speech-to-text transcription. Its goal is to teach computers to understand human speech and text, similar to how another human being would. NLP focuses specifically on training machines to interpret text, speech, meaning, sentiment, intent, and context. Recent advances in NLP have allowed speech-to-text applications to proliferate and reach the adoption rates we see today.
Real-World Uses of Text-to-Speech Today
As speech-to-text applications became available to consumers in the 1990s, it initially remained essentially a specialty service. Its use was generally reserved for government and private sector businesses for data recording/archiving purposes.
It's a very different story today, where pretty much anyone with a smartphone and an internet connection can make use of speech-to-text applications in one form or another.
As the tech's adoption grew and its users became more and more diverse, so did the use of the technology. Today, speech-to-text software is used across industries and has more applications than ever.
We can broadly group how speech-to-text software is used today into the following four categories:
- Customer Service: It's no secret that you may have spoken to a bot the last time you phoned a company's Support department. Many businesses use AI assistants as their first line of support to cut costs and improve the customer experience.
- Content Search: With voice assistants on every smartphone, the increase in voice search has been colossal. And today's speech-to-text applications can recognize words and complete sentences to provide accurate and relevant results quickly.
- Content Consumption: With streaming services taking over traditional television, the demand for digital subtitles has exploded. Real-time captioning represents a huge market as content is streamed worldwide to viewers speaking different languages simultaneously.
- Electronic Documentation: Live transcription remains one of the top uses of speech-to-text applications. Everyone from doctors and lawyers to government agencies all gain from efficient record-keeping. And live transcription makes that much easier. Many businesses also use the tech to extract valuable information from remote meetings and video conferences.
The Challenges of Speech-to-Text
While the quality of speech-to-text applications has never been better, there are still some areas where the tech doesn't perform optimally. We have an average error rate of 5% today. And while we can expect that number to shrink with every passing day, below is a shortlist of some of the most prevalent factors that can cause a bit of a struggle for speech-to-text applications.
Accents and Dialects: People who speak the same language will have different accents, and specific populations will have their own dialect of a given language. That sometimes makes it difficult for actual human beings to understand each other. It's no different for voice assistants. However, the rapid advances in NLP lead us to believe that this won't remain a challenge for long.
Context: Things like homophones (words that sound the same but have different meanings), local expressions, or sarcasm, for example, can be challenging for AI because it's a machine that lacks the cultural awareness of humans. However, we're getting better and better at building robust language models and training the machines on problematic words and expressions in context.
Code-Switching: Code-switching means using multiple languages within a conversation. And it's more common than you might think. There are many multilingual communities where people use multiple languages in a single conversation. That introduces additional complexities for the language model that needs to handle "random" switches in lexical and grammatical patterns as users switch from one language to another (sometimes within the same sentence!)
Wrap Up
So that's the state of speech-to-text applications today. They've come a long way and will likely be with us for a long time. As their capabilities improve and voice assistants are able to accomplish more and more valuable tasks, I think we'll be seeing (hearing?) the use of the tech in more and more places. And as natural language processing ups its game over time, we may well reach the point where its accuracy can legitimately be taken for granted.
Keep your ears open!
About Modev
Modev was founded in 2008 on the simple belief that human connection is vital in the era of digital transformation. Today, Modev produces market-leading events such as VOICE Global, presented by Google Assistant, VOICE Summit, and the award-winning VOICE Talks internet talk show. Modev staff, better known as "Modevators," include community building and transformation experts worldwide. To learn more about Modev, and the breadth of events offered live and virtually, visit modev.com.